Stochastic Resetting for Enhanced Sampling
Combining stochastic resetting with Metadynamics to speed-up molecular dynamics simulations
Molecular dynamics (MD) simulations are a powerful tool, providing microscopic insight into the properties of chemical and physical systems. However, their atomic temporal and spatial resolution limits MD simulations to processes shorter than a few microseconds. Longer processes, such as protein folding or crystal nucleation and growth, cannot be sampled efficiently by standard MD simulations. This "timescale problem" is an open challenge for theoretical chemistry.
Over the years, different enhanced sampling methods have been developed to overcome the timescale problem. Many of them rely on identifying collective variables (CVs) that capture the slowest modes of the system. Metadynamics (MetaD) is one popular method that uses an adaptive, external bias potential to enhance the sampling along the chosen CVs. It involves the ongoing deposition of bias at previously visited regions of the CV space. This technique is demonstrated in Video 1, where a particle is pushed to cross an energy barrier along the CV. MetaD can accelerate processes by orders of magnitude but is highly dependent on the quality of the CVs. Unfortunately, despite recent progress, finding good CVs in complex systems is also an open challenge.
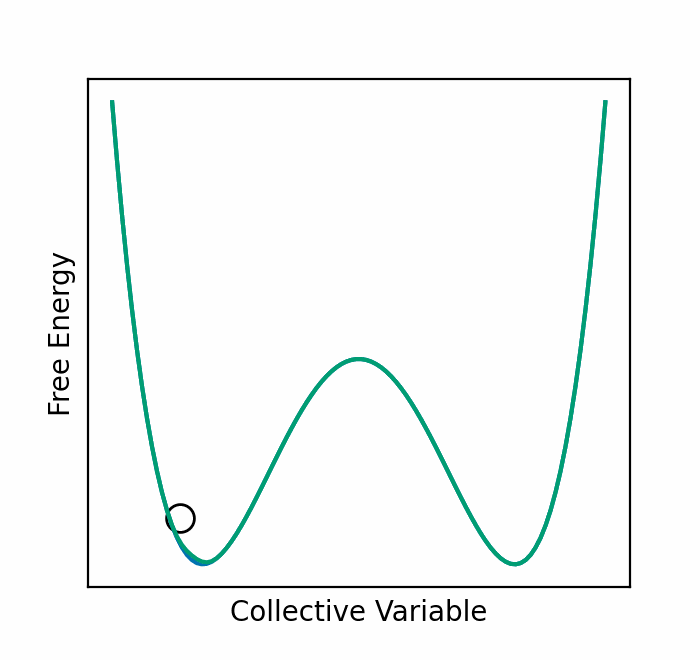
We recently presented an alternative, CV-free approach for enhanced sampling using stochastic resetting (SR): a procedure in which stochastic processes are stopped randomly and restarted, subject to the resampling of independent and identically distributed initial conditions. Resetting received much attention recently and was shown to expedite a wide range of first-passage processes. Video 2 demonstrates this for a diffusing particle in a two-dimensional system. The particle reaches the target upper well faster, on average, if we occasionally restart the simulation, returning it to its initial position. In a recent paper, we showed that SR can also accelerate MD simulations by up to an order of magnitude. However, it may not be beneficial for all systems.

Resetting was previously shown to be particularly efficient for flat landscapes. Interestingly, MetaD is designed to overcome energy barriers by flattening the free energy surface (FES), as demonstrated in Figure 1. The unbiased FES of the system has a high barrier and narrow basins (solid line). Resetting is not necessarily expected to accelerate the transitions between the basins in this case. However, the external bias of MetaD produces an effective flatter FES with a lower barrier and broader basins (dashed line). This means that while MetaD accelerates the transitions, it also encourages excessive sampling of high-energy regions of phase space away from the barrier. The poorer the CV, the likelier it is for the system to "get lost" in these undesired regions. We noticed that this is exactly the scenario where SR thrives. This observation motivated us to study the combination of MetaD with resetting.

In this paper, we show how MetaD and SR complement each other. Their combination enables greater accelerations and/or better kinetics inference than either approach separately. We present a model system where SR further accelerates MetaD simulations, even when using the optimal CV. In another model system, we show that restarting MetaD simulations using suboptimal CVs gives similar accelerations to using the optimal CV. This suggests that resetting can serve as an alternative, or complementary, method to improving existing CVs. We show this holds also for two molecular systems: For a small molecule (alanine tetrapeptide), resetting accelerates simulations using a poor CV by more than two orders of magnitude. For the folding of a mini-protein in water (chignolin), adding SR to MetaD simulations using a naive, suboptimal CV gives similar accelerations to those obtained when using a more sophisticated CV, based on dimensionality reduction algorithms.
Lastly, we suggest a new kinetics inference procedure for simulations combining MetaD and SR. We employ it to estimate the mean first-passage time between two metastable states of the alanine tetrapeptide molecule, from simulations employing a suboptimal CV. Our method results in a better tradeoff between speedup and accuracy, compared to a popular inference method.
Resetting is a computationally cheap tool, that can be employed straightforwardly in existing MD codes. We believe that additional enhanced sampling methods that flatten the FES would also benefit from it. Furthermore, we supply a simple procedure to assess if it would be beneficial, based on a limited number of available trajectories, showing one transition each. We hope to see more exciting combinations and applications of SR and enhanced sampling soon!
References: